Matrices
1. A = [1 2; 3 4; 5 6]

2. size(A)
size of matrix

3. size(A,1)
number of rows
ans = 3
4. size(A,2)
number of columns
ans = 2
5. A(3,2)

ans = 6
6. A(2,:)
every element along row 2

7. A(:,1)
every element along column 1

8. A([1 3],:)
every element along rows 1 and 3

9. A(:,2) = [10; 11; 12]
replace column 2 with new elements

10. A = [A, [100; 101; 102]]
append new column vector to the right
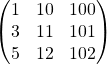
11. A(:)
put all elements of A into a single vector
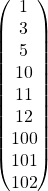
12. A = [1 2; 3 4; 5 6]
B = [11 12; 13 14; 15 16]
C = [A B]
concatenating A and B
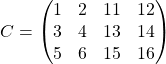
13. C = [A; B]
putting A on top of B
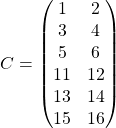
14. v = [1 2 3 4]

15. length(v)
length of vector v
ans = 4
Loading files
1. path: pwd
shows where Octave location is
2. change directory: cd '/Users/eugene/desktop'
3. list files: ls
4. load files: load featuresfile.dat
5. list particular file: featuresfile
6. check saved variables: who
7. check saved variables (detailed view): whos
8. clear particular variable: clear featuresfile
9. clear all: clear
10. restrict particular variable: v = featuresfile(1:10)
only first 10 elements from featuresfile
11. save variable into file: save testfile.mat v
variable v is saved into testfile.mat
12. save variable into file: save testfile.txt v -ascii
variable v is saved into text file